Empowering Artificial Intelligence Techniques with Soft Computing of Neutrosophic Theory in Mystery Circumstances for Plant Diseases
Keywords:
Neutrosophic theory, Deep Learning, plant diseaseAbstract
Plant diseases are one of the factors that lead to yield and economic losses, which have a direct effect on
national and international food production systems. One of the most essential ways to avoid agricultural
product loss or reduction in amount is to diagnose plant diseases promptly and accurately. Hence, the
diagnosis process for plants is crucial and should be conducted accurately. Moreover, this study focuses
on this process by constructing an Artificiality Diagnostics Framework (ADF) to serve the study’s objectives
which entailed conducting diagnosis for plants in a professional and precise manner over uncertain
environments. Thus, neutrosophic theory is considered the principal ingredient in our ADF. Due to the
ability of neutrosophic to divide images into Truth (T(, Falsity(F), and Indeterminacy (I). Also, deep
learning (DL) is considered another principal ingredient in treating vast samples of datasets. Our
comparative analysis of the leaves of potatoes is conducted whether leveraging neutrosophic and without
utilizing Neutrosophic. ResNet50, ResNet152, and Mobile Net are the principal ingredients for the training
dataset. The findings of implementing these networks indicated that ResNet50 achieved the highest
accuracy of 0.915 in the T domain, ResNet152 achieved the highest accuracy of 0.905 in the True(T) domain,
and Mobile Net achieved the highest accuracy of 0.915 in Truth(T) domain. Accuracy of 0.863 in
Indeterminate(I).
Downloads
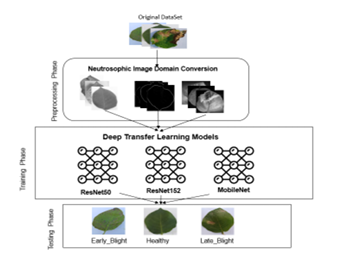
Downloads
Published
Issue
Section
License
Copyright (c) 2024 Neutrosophic Sets and Systems

This work is licensed under a Creative Commons Attribution 4.0 International License.