Enhanced Federated Learning Framework based on Deep Learning and Neutrosophic Set for Android Malware Classification
Keywords:
Malware detection; Deep Learning; Federated Learning; Interval Valued Neutrosophic SetsAbstract
Malware detection is one of the critical tasks of cybersecurity, especially considering the growing
popularity of mobile devices. The integrity and security of mobile ecosystems rely on the capacity to
identify malware quickly and precisely, as security hacks against these devices become increasingly
frequent. Due to the requirement for massive volumes of data aggregation, traditional centralized machine
learning (ML) techniques for malware detection face challenges with data sharing, computational
complexity, and privacy. This research addresses these challenges by proposing a novel model, called
"CNN-Fed", which is based on Convolutional Neural Networks (CNN) with Federated Learning (Fed). The
main goal of this work is to create a global classifier for Android malware detection that is highly accurate
and does not require centralised data aggregation. Using four benchmark datasets—Drebin, Malgenome,
Kronodroid, and Tuandromd—the CNN-Fed model is trained across many clients in a federated setting
under the suggested architecture. Then we use multi-criteria decision-making (MCDM) methodology to
evaluate the number of clients and number of rounds. We use two MCDM methods such as Entropy
methodology to compute the criteria weights and the CoCoSo methodology to rank the alternatives. We
use the interval-valued neutrosophic sets (IVNSs) to deal with uncertainty and vague information. We use
five criteria such as accuracy, F1-score, precision, recall, and FP and six of alternatives refer to the number
of clients and number of rounds. The results show the best alternative has a larger number of clients and
rounds.
Downloads
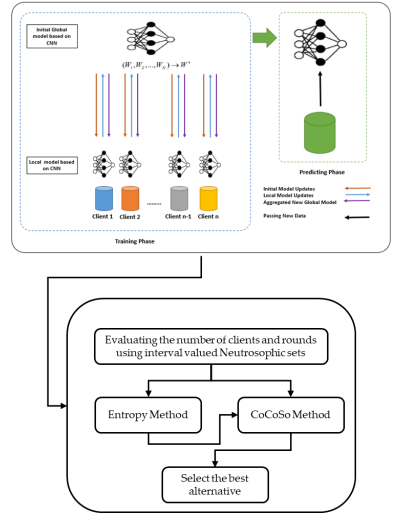
Downloads
Published
Issue
Section
License
Copyright (c) 2025 Neutrosophic Sets and Systems

This work is licensed under a Creative Commons Attribution 4.0 International License.