Neutrosophy, Causal AI, and Web3: combo for complex decision-making
Keywords:
Neutrosophic Causal AI, do-operator, uncertainty modeling, causal inference, Web3 applicationsAbstract
This article introduces Neutrosophic Causal AI, a novel framework that integrates neutrosophic logic with structural causal models to enhance decision-making under uncertainty. While traditional Causal AI is effective at identifying cause-and-effect relationships, it assumes a level of precision rarely found in complex, real-world systems. By incorporating degrees of truth (T), indeterminacy (I), and falsity (F), Neutrosophic Causal AI extends causal inference to accommodate ambiguity, contradiction, and incomplete data. The proposed framework formalizes the neutrosophic do-operator and adapts Judea Pearl’s structural causal models to a neutrosophic context, allowing for more nuanced intervention analysis and counterfactual reasoning. Through illustrative examples and a simulation-based approach, the article demonstrates how this method improves transparency and epistemic robustness in decision systems. Special attention is given to applications in Web3 environments, where decentralized governance, smart contracts, and autonomous decision-making require high levels of reliability and trust. Neutrosophic Causal AI thus emerges as a critical tool for building intelligent systems that reflect the complexity of social and digital ecosystems, providing a bridge between computational logic, causal analysis, and real-world ambiguity.
Downloads
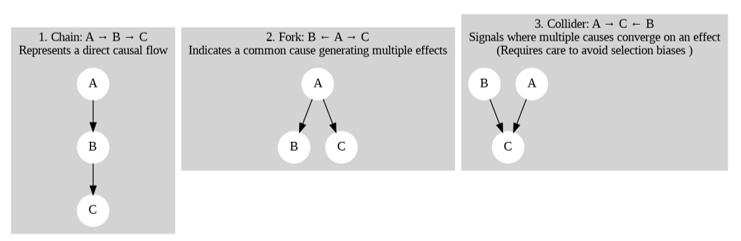
Downloads
Published
License
Copyright (c) 2025 Neutrosophic Sets and Systems

This work is licensed under a Creative Commons Attribution 4.0 International License.