Stock Price Predictions with LSTM-ARIMA Hybrid Model under Neutrosophic Treesoft sets with MCDM interaction
Keywords:
MCDM, TOPSIS, AHP, Treesoft sets, deep learning, LSTM, ARIMA, Stocks, equitiesAbstract
The stock market is regarded as volatile, complex, tumultuous, and dynamic.
Forecasting stock performance has proven to be a challenging endeavour due to its increasing
need for investment and growth prospects. At the forefront of machine learning, deep learning
models facilitate the straightforward and efficient exploration and identification of optimal
stocks, the hybrid forecasting models (LSTM and ARIMA) are used in the prediction of stock
increase. This paper incorporated the MCDM technique to determine the optimal stocks for
investment. The Analytic Hierarchy Process (AHP) is used to assign weights to various
financial factors. These weights are then used by the Technique for Order Preference by
Similarity to Ideal solution (TOPSIS) technique, which is a component Multi Criteria Decision
Making method (MCDM), to compute and rank the optimal stocks for investment. Stock
analysis involves considering numerous criteria and sub-criteria, which might lead to an
unsuitable answer. To address this uncertainty, we utilize Neutrosophic Treesoft sets, which
primarily handle numerous criteria, sub-criteria, and an increased number of sub-sub-criteria.
Given a larger number of criteria, we will be capable of providing a precise solution to the
problem. Furthermore, the definitions of fuzzy treesoft sets and neutrosophic treesoft sets have
been presented for the first time. A plotly graph is generated to compare the real and projected
stock prices for all the equities. All these are implemented using the program language python,
which seems to be simple and easily understandable when compared to the other programming
languages like Julia, MATLAB and so on. This hybrid methodology facilitates the forecast of
stock prices, the ranking of stocks based on several financial and non-financial factors using
AHP and TOPSIS, and the visualization of the outcomes.
Downloads
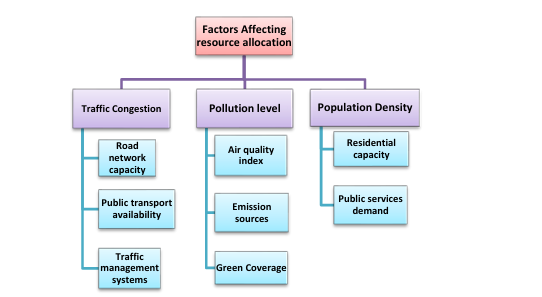
Downloads
Published
Issue
Section
License
Copyright (c) 2025 Neutrosophic Sets and Systems

This work is licensed under a Creative Commons Attribution 4.0 International License.