Hybrid Neutrosophic Deep Learning Model for Enhanced Arabic Handwriting Recognition
Keywords:
Handwritten Character Recognition; Deep Learning; Arabic Natural Language Processing; Optical Character Recognition; Neutrosophic SetsAbstract
Recognizing handwritten Arabic characters poses a significant challenge due to the
complexities of the cursive script and the visual similarities between characters. While deep learning
techniques have shown substantial promise, advancements in model architectures are essential to
further enhance performance. Neutrosophic Sets (NS) have demonstrated their potential in
improving classification models by effectively handling indeterminate and inconsistent data. This
paper introduces a novel approach that integrates Neutrosophic Sets with a hybrid deep learning
model, combining Convolutional Neural Networks (CNNs) with Bidirectional Recurrent Neural
Networks (Bi-LSTM and Bi-GRU). This integration allows for the extraction of spatial features and
modeling of temporal dynamics in handwritten Arabic text. Experiments conducted on the Hijjaa
and AHCD datasets revealed that the NS_CNN_Bi-LSTM model achieved an accuracy of 92.38% on
the Hijjaa dataset, while the NS_CNN_Bi-GRU model attained 97.38% accuracy on the AHCD
dataset, outperforming previous deep learning approaches. These results highlight the significant
performance improvements achieved through advanced temporal modeling and contextual
representation, without the need for explicit segmentation. The findings contribute to the ongoing
development of highly accurate and sophisticated deep learning systems for Arabic handwriting
recognition, with broad applications in areas requiring efficient extraction of text from handwritten
documents.
Downloads
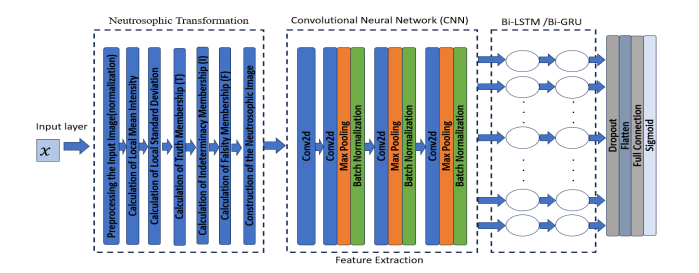
Downloads
Published
Issue
Section
License
Copyright (c) 2024 Neutrosophic Sets and Systems

This work is licensed under a Creative Commons Attribution 4.0 International License.