Neutrosophic computational model for identifying trends in scientific articles using Natural Language Processing
Keywords:
Natural Language Processing, Neutrosophic Logic, Trend Analysis, Information Extraction, Summary Validity, Neutrosophic Computational ModelAbstract
This study presents an innovative neutrosophic computational model for identifying trends in scientific articles through Natural Language Processing (NLP). The primary objective was to develop a system that could effectively analyze a large body of academic literature and extract relevant summaries that capture the most significant themes and findings. Advanced NLP techniques were employed to extract, process, and synthesize key information, enabling a deeper understanding of emerging trends. Furthermore, the model was designed to evaluate the relevance and validity of the generated summaries, ensuring that the information presented is accurate and useful for researchers and practitioners. The results demonstrate that the system is not only efficient in generating coherent summaries but also facilitates the identification of critical themes in scientific research, potentially guiding future research and applications in the field. Thus, this model is established as a valuable tool for the academic community, promoting more agile and effective access to relevant information in a constantly evolving scientific context.
Downloads
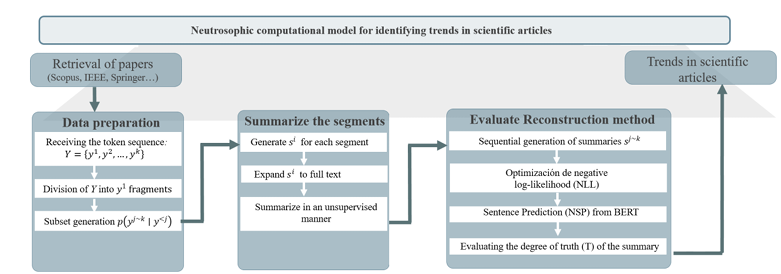
Downloads
Published
License
Copyright (c) 2025 Neutrosophic Sets and Systems

This work is licensed under a Creative Commons Attribution 4.0 International License.